CCPS Vorträge: Abschlussarbeiten
Mittwoch, 29. Mai 2024, 14:00 und 15:00 h
29.05.2024
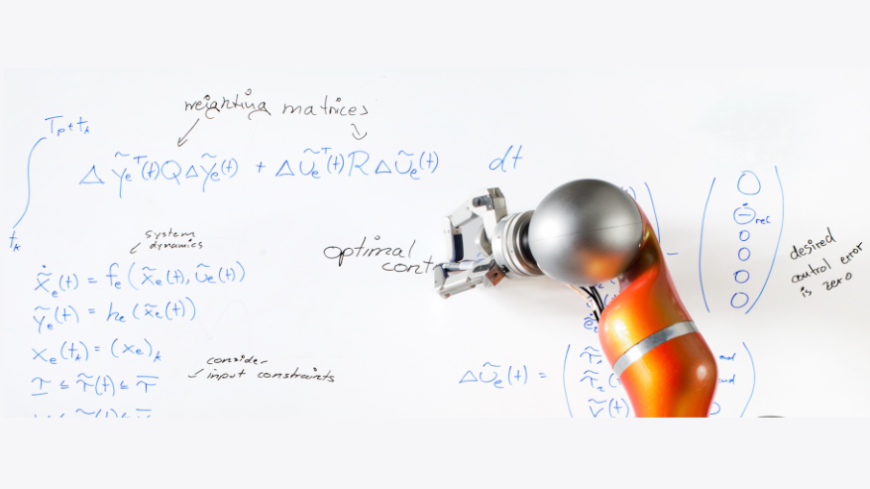
Gäste sind herzlich willkommen. Es finden folgende Vorträge statt:
14:00: Handong Yao (Master Thesis)
„Compensation of Delays in Nonlinear Model Predictive Control Using NLP Sensitivity Analysis“
Betreuer: Lukas Theiner, M.Sc., und Andreas Höhl, M.Sc.
Raum S3|10 406A
oder via Zoom: https://tu-darmstadt.zoom-x.de/j/66153197176?pwd=WWVGOWVDTkR2T1ZyK3VsUlQzK2lDQT09
Meeting ID: 661 5319 7176
Passcode: 399544
15:00: Caio Freitas (Master Thesis)
Graph Diffusion for Imitation Learning in Robotics
Betreuer: Ali Younes, An Thái Lè, Prof. Georgia Chalvatzaki (FB 20) und Maik Pfefferkorn
Raum S2|02 E202
oder via Zoom: http://talks.robot-learning.net/
Abstract: In the field of robotics, imitation learning (IL) has emerged as a powerful paradigm, enabling machines to learn increasingly complex behaviors from human demonstrations. While conventional IL methods require careful engineering of observation and action spaces, recent advancements in diffusion models and graph neural networks (GNNs) offer promising methods for more flexible and general learning approaches. This thesis explores the fusion of these advancements by proposing a novel framework that leverages diffusionbased graph generation for IL in robotics. We introduce two graph diffusion policies for IL: GraphDDPM, based on denoising diffusion probabilistic models, and ARGG, which explores the autoregressive graph generation framework. These aim to expand the applications of such models to robotic action generation tasks. Our approaches differ from existing methods by incorporating joint-level proprioception and action representation, treating the environment and manipulator as graphs, and operating directly in the graph domain to utilize structural information. We conduct comprehensive experiments to validate our approaches and their qualities, comparing its performance against traditional IL methods and contemporary state-of-the-art techniques. Additionally, we discuss limitations and future improvements to the developed framework, aiming to incentivize future research applying such models in robotics. Through this research, we aim to bridge the gap between diffusion-based graph generative models and robotics, offering insights into the potential of this approach for future advancements in robotic learning and autonomy.