Neuer Artikel: „Geometric Data-Driven Dimensionality Reduction in MPC with Guarantees“
Roland Schurig, Andreas Himmel, Rolf Findeisen
14.12.2023
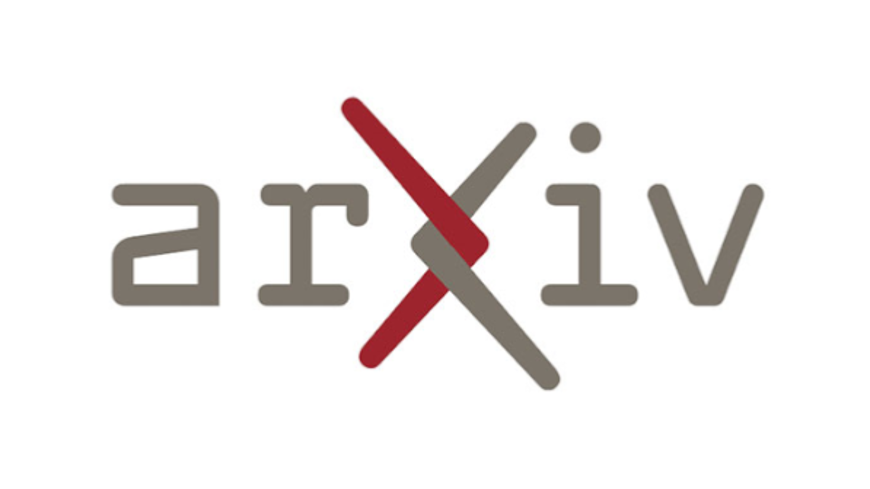
Geometric Data-Driven Dimensionality Reduction in MPC with Guarantees
Abstract
We consider the problem of reducing the dimension of the discrete-time optimal control problem that is solved repeatedly online in model predictive control. We show that a reduced-order scheme, which solves the optimization problem in a low-dimensional subspace, inherits the stability and recursive feasibility properties from the original formulation. We introduce a necessary and sufficient condition for initial feasibility and incorporate that in the subspace design. Finally, we use concepts of optimization over Riemannian manifolds to compute a subspace that provides optimal representations for a set of pre-defined high-dimensional optimizers under the initial admissibility constraint.