New Article on Functional High-probability stable Gaussian process-supported model predictive control for Lur’e systems
Hoang Hai Nguyen, Maik Pfefferkorn, Rolf Findeisen
2022/06/30 von Ilse Brauer
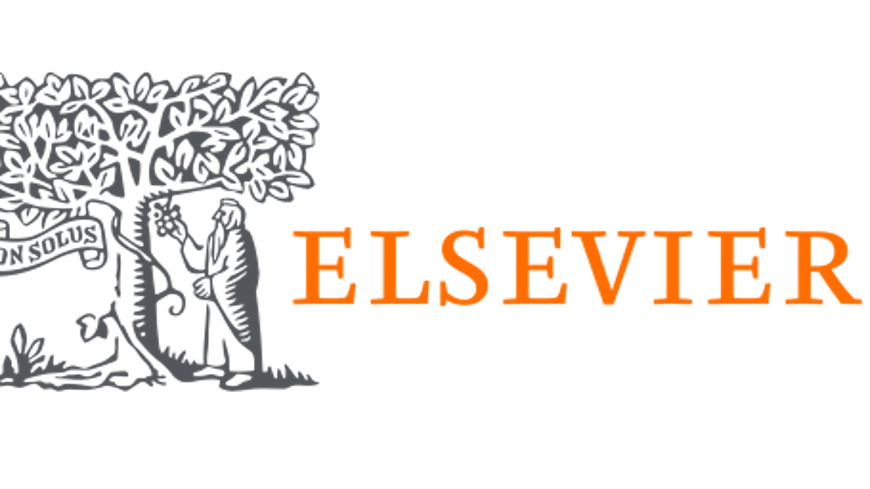
High-probability stable Gaussian process-supported model predictive control for Lur’e systems
Abstract
Machine learning methods, like Gaussian process regression, allow improving the performance of model-based control methods, such as model predictive control. They can, for example, be used to improve the prediction quality of the used model of the system, learning the uncertain system part. However, fusing model-based approaches with machine learning methods raises the question of stability and performance guarantees. This work considers Gaussian process regression to learn uncertain system parts for nonlinear Lur’e type systems. More precisely, the Gaussian process is used to learn the nonlinearity of the system. Based on the learned model, it is shown how to compute suitable terminal regions to guarantee closed-loop stability. To do so, reproducing kernel Hilbert space membership of the learned function is exploited to compute high-probability sector bounds for the nonlinearity. From the obtained bounds, a high-probability terminal region that guarantees closed-loop stability is derived by methods from robust control theory. A flexible joint robotic arm example underlines the effectiveness of the approach.
